It’s no secret that lidar sensors that help autonomous cars detect surrounding objects are expensive, often costing more than the cars themselves. It’s also no secret that people have questioned their effectiveness in certain road conditions. And while car manufacturers are still in the process of getting self-driving cars into real-life conditions, there’s an ongoing search for possible alternatives for lidar. Inexpensive cameras could be the answer, although this would require the production of real-time 3D models of their surroundings to allow autonomous vehicles to safely navigate the streets. Additionally, a translation from 2D information to 3D objects would be necessary, as cameras acquire only 2D images from which separate objects have to be distilled.
A new paper from Mapillary, shared at an international conference on computer vision, demonstrates advances in existing methods for 3D object recognition in 2D images using AI. This is good news for autonomous car manufacturers who are turning to inexpensive cameras instead of lidar, with the goal to enable safe autonomous navigation.
Estimating 3D bounding boxes
Mapillary’s platform consists of continuously updated 2D street-level imagery from all over the world. This platform has enabled them to automate object detection in 2D imagery and create traffic sign data for their customers. They have also created 3D models from the same 2D street imagery, using Structure-from-Motion (SfM) techniques to match thousands of points between different images in the same location.
However, performing 3D object detection in 2D images has proven to be more complicated in computer vision, explains Peter Kontschieder, who is Director of Research at Mapillary.
“What we’re trying to do is to estimate the 3D bounding boxes of objects in a standard RGB image, as we are interested in localizing the content inside street level imagery, such as cyclists, cars, pedestrians and the like. The problem is it’s not really possible – in a mathematical definition – to extract 3D bounding boxes based on a single image of an ocular image view”.
Using a machine learning approach, Mapillary developed a model that creates 3D bounding box around objects in a 2D image, consisting of eight corners, by adding information about the 3D extent of objects to an existing 2D bounding box. This 3D extent describes the depth of the bounding box, which is basically the distance to the camera to the center of the 3D bounding box. A second group of parameters try to define the size, height, width and depth of the box. All these parameters define a well-lined box to show how the object is located with respect to the camera.
Mapillary’s research was presented as a paper titled “Disentangling Monocular 3D Object Detection” during the 2019 International Conference on Computer Vision held in Seoul, Korea.
Topping lidar-based approaches
Mapillary’s actual contribution with this the paper explains that disentangling all the different levels of predictions results in a smoother optimization: not only is it much faster, the final result is also significantly better than what others have been doing when they tried to solve the same problem. In addition to this, the company’s researchers have participated in multiple conferences and workshops this year showing that their approach as a serious alternative to existing solutions for object detection from single images: at the latest edition of the Conference on Computer Vision and Pattern Recognition (CVPR), for example, they even topped two lidar-based approaches.
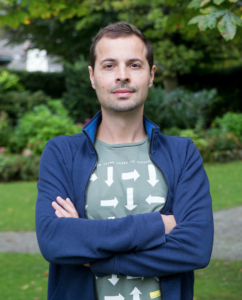
Peter Kontschieder, Director of Research at Mapillary
Asked what Mapillary’s object recognition approach means for the current use of lidar in autonomous driving, Kontschieder answers that the first generation of autonomous vehicle manufacturers will still be operating with lidar-based systems. This is mainly as a way to overcome the mathematical problem stated before, as lidar provides an active sensor that measures the distance of objects which is an important feature for doing 3D object detection in a self-driving context.
“What we’re seeing now is that people try to exploit different sensors to overcome the problems of lidar, such as the reflectance of certain objects. However, the long-term goal is how to move forward with only passive camera-based settings, meaning cheaper setups with maybe some less cheap sensors for interesting complimentary features, such as lidar or radar”.
Moving forward, the industry tries to solve the many problems with regards to autonomous driving using street level data and train models to deal with high-variety data.
“At ICCV for example, we discussed the current existing problems with regards to autonomous driving with other scientists, such as poorly illustrated areas that result in poor image quality, or crowded areas with rare objects that are not often seen in the dataset. One of the major assets of our platform is that we have a broad body of data with data from all over the world, which we can expose the algorithms to during training. The scale that we’re able to provide gives us a head start in the market”.