Conversations around artificial intelligence have broken out of the technology world and into everyday life, with generative AI serving as the main focus in the mainstream. That said, AI of all kinds is seeing a boom in interest and investment, leading to better workflows across industries. Geospatial is no exception, as we covered in a recent report discussing AI’s impact on the industry. Those largely focused on areas like insurance and city planning, but the combination of geospatial data and artificial intelligence can have big impacts in ESG initiatives and other people-focused applications.
Specifically, one area where we have seen a relatively recent application of AI and geospatial data is in disaster preparation and response in India, thanks to an AI model called Sunny Lives developed by Gramener in partnership with SEEDS and Microsoft. Sundeep Reddy Mallu is the head of Analytics and ESG with Gramener, and he recently took some time to speak with Geo Week News about the Sunny Lives model, how it works, and what is needed to continue this momentum.
Before getting to the model, though, it’s worth taking a second to talk about Mallu’s journey, as well as his title at Gramener. It’s a unique title that we don’t see very often, combining a highly technical field like analytics with one which is sometimes seen as more of a “soft science” in ESG. With the aforementioned explosion of AI, though, as well as the increasing motivation of companies to address ESG concerns – due both to those concerns increasing in scope as well as regulatory requirements – I suspect this kind of combination will only become more common.
As for Mallu, he acknowledged it’s not common but explained his path to the role. He notes that when he started with Gramener, the company had fewer than 50 employees, compared to the 250 employees today. As such, while he started on the data science side, “as the company grew,” Mallu told Geo Week News, “I started taking more responsibilities. I continued wearing my analytics hat, and also picked up the role for ESG. I grew along with the organization with more responsibility.”
Mallu also talked about his past in relation to Sunny Lives, which was developed by Gramener in partnership with SEEDS and Microsoft, focusing on preparing at-risk residents who could be impacted by cyclones in India. Mallu grew up in a farming community in a “drought-prone” area in the nation. In 2019, he noticed an abundance of rain fall, which he says caused him to “wake up to the realization of how bad things are getting” with respect to climate change. From that point, he, and by extension Gramener, began looking further into solutions to address those affected by more erratic climate events.
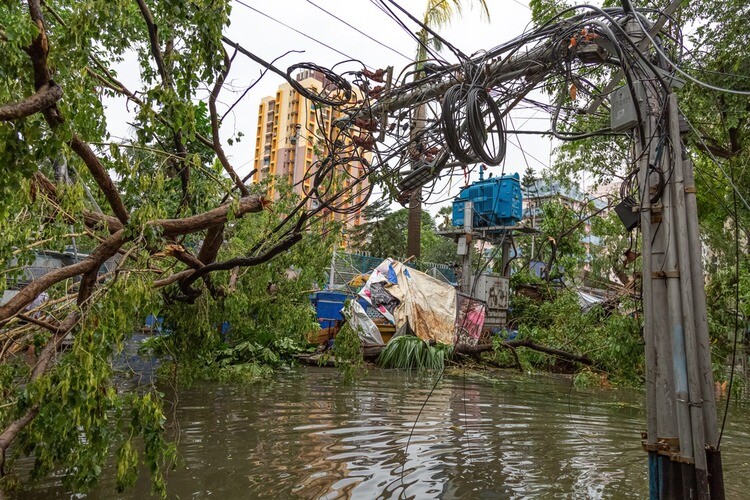
That brings us to the Sunny Lives model, which they started working on in 2020. Mallu said, “Every year, most of the state governments spend anywhere between 6,000 and 7,000 Indian rupees on disaster relief,” illustrating how much of a problem this is for the most populous nation in the world. SEEDS, meanwhile, is an Indian organization founded in 1994 which focuses on disaster relief efforts throughout the nation. They worked with Gramener to try and determine a preparation strategy to make these recovery efforts both more efficient and more cost-effective.
Mallu said, “We know the cyclone pattern is consistent along the coastline, and we know the low-lying areas are most affected more often than not.” From there, it was about finding the most common factors which determine how much damage a house takes on due to a cyclone. That led to the foundation of this model, which takes in satellite imagery and identifies different types of rooftops in areas which can be affected by a cyclone, as that is an indication of how well a house can hold up to a storm and flooding. Using this data, as well as other geospatial information like nearby waterways and topography, houses in an area are given a risk score of one through five, and houses on the higher end of that scale are prioritized for evacuation warnings and other preparation efforts.
Initially they ran four pilots – with initial funding from Microsoft’s AI for Humanitarian Action program– across different cyclones in different parts of Southern India along the coastline. Mallu said, with the help of the Sunny Lives model, “SEEDS volunteers on the ground were able to go to houses that were more susceptible and give them at least a two-day head start, which in turn helps people safeguard their assets and property and evacuate.” They also ran post facto ground validation, finding their model achieved 70 to 75 percent accuracy in predicting the risk score for individual houses. That’s not a number which jumps off the page, but as Mallu points out, “Compare that with no warning at all.” It can be the difference between life and death.
In addition to cyclones, and the subsequent flooding, Mallu notes that they are looking to expand the usage of their model into other disasters as well. For example, the Sunny Lives model can also be applied to earthquakes, although weighting different metrics and data types differently and looking at different things. They look again at topography and elevation, as well as things like soil type to determine landslide risk.
Interestingly, the model has also been used for heat waves, which is already a problem in India and only figures to get worse as climate change progresses. Mallu does point out that people are less likely to respond to impending heat risk than something like a flood, but this information is still crucial. The Sunny Lives model takes into account an urban area’s layout and determines “urban heat islands” to figure out vulnerable areas and where things like water stations should be set up. He even notes that urban planners around the world have utilized this model for their work, such as the city of Calgary in Canada, determining how adding a new building to an area will affect heat in the space.
While they’ve already seen results with this model, Mallu points out a couple of things that are needed to continue developing and hopefully scale it for global purposes. The first is more accurate and timely information about where exactly a cyclone is projected to make landfall. Mallu said that’s at “the top of his list” to solve for, and that they “need more partnerships to get more compute in place.” He continued, “anything to do with better forecasting simulations requires heavy, heavy computing.” He also points to the cost of recent and accurate satellite imagery, which he says right now costs about $14 per square kilometer.
These kinds of solutions are only going to become more necessary as the effects of climate change become more pronounced, but fortunately the rapid growth of artificial intelligence should help keep pace. Gramener’s Sunny Lives model is one example of how this can happen, helping save lives with the use of geospatial data.