Contributed by Dr. Jun Pei, CEO and co-founder of Cepton
Artificial Intelligence (AI) has dominated recent news cycles, with scary headlines citing the “end of civilization” and speculating about what these supercomputers could do for the future of our society. It's true we don’t know what lies ahead with this next revolution of AI intelligence, but what we do know is that the data sets being used to train AI-based systems have a tremendous impact on what they spit out.
As an engineer and technologist, I’ve always been fascinated with how things go together – what makes us tick. AI has a tremendous ability to digest and regurgitate “somewhat reliable” information; yet, it makes me think about the data going in, what is being swallowed up and digested to make AI work and able to “predict” outcomes, responses and behaviors.
We must ask ourselves: Are we feeding AI-based systems data that properly represents all populations, genders and ethnicities? If not, it may create an innate bias in the AI technology we are looking to rely on, proliferating generational prejudice and favoritism unintendedly.
Users recently searched AI platforms Craiyon, Mindjourney and Stable Diffusion with the prompt “office boss future,” and 100 percent of the images produced were of white males. Other prompts resulted in similar outcomes. Additionally, a Pro Publica report found that a U.S. court’s AI risk assessment technology was flagging black defendants as likely to reoffend at almost twice the rate as white defendants. Similarly, AI systems used by financial lenders were found to regularly deny housing and refinance opportunities, as well as charge higher rates, to people of color.
This presents an incredible risk to our society. As AI technology is being used in autonomous vehicles, surveillance, and crowd management applications to generate analytics and enable intelligent decisions, biased data from cameras, cellphones and other devices might lead to highly inaccurate results.
Lidar, a technology which uses light pulses to identify objects and distances, can help minimize bias by providing impartial, behavior-based data. Instead of who it sees, lidar focuses on what it sees – think about movement patterns, how much time spent in a zone, and proximity to sensitive area.
One alarming example pertains to assisted and autonomous driving. If the pedestrian data used in the technology that drives our cars is only trained on camera images of adult white males – be it a real person or a dummy – the system’s capabilities of identifying other races, women, and children are compromised, and it is likely to fail at detecting all pedestrians. When it comes to pedestrian safety, biometric information would be the least important data to collect and on which to base an automated safety feature.
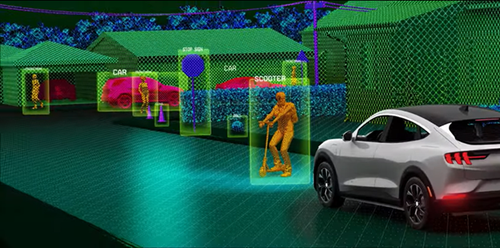
For the past seven years, I have been focused on developing lidar solutions for everyday automotive use. A fundamental requirement for any automotive-qualified software system is that it must be a deterministic system, meaning for a given input the output should always be the same. It is very easy to understand for wired control for brakes and steering wheel – every time you press the brake and send the signal to the brake actuator, it makes the exact same action.
When it comes to AI systems, it is never 100% deterministic in pretty much all the scenarios. The automotive industry is still struggling to understand and utilize the system without incurring unnecessary liability. By reducing bias introduced by unnecessary biometric data, lidar makes the system more deterministic on the software level, providing an additional layer of certainty to the vehicle’s AI-based perception platform and making it more readily adoptable for mass-market applications.
Similarly, in security and facility management applications, data based on photo and video footage marked up with biased conclusions could lead a person to be identified as a threat based on their race, gender, age, or outward appearance. Because lidar captures and outputs anonymous data, it eliminates this possibility. When used in surveillance, lidar provides such accurate 3D imaging that it effectively reduces false positives and false negatives of alarming events; it flags suspicious activities purely based on behaviors, based on which camera footage can then be used for further confirmation. This helps reduce unnecessary staff intervention in the initial stages, resulting in lower operational costs and mitigating unwanted bias. After all, let’s face it, we humans tend to form unconscious judgements as well.
As retail stores and public venues increasingly rely on crowd analytics to enhance the customer experience, lidar also helps solve for data biases based on exclusion, in addition to protecting people’s privacy. For example, if a concert venue measures activity based on Bluetooth and Wi-Fi connectivity, they are ignoring those who do not carry a smart phone with them, such as children, seniors, physically or financially constrained attendees, or those who simply wish to spend some phone-free time. But do those people still need to be counted for queue management or restroom sanitization? Yes, and lidar would track their movements accurately to enable privacy-sensitive analytics in real time.
Integrating lidar into training data for AI will make it far easier and cheaper to address and ensure the data going into smart systems are less biased and more accurate, as opposed to retroactively identifying errors once AI technology has further evolved. Lidar’s high accuracy, 24/7 availability, intelligence, and anonymity make it a valuable and powerful technology to rely on as we advance into a future of cleaner, smarter data.
Dr. Jun Pei, CEO and co-founder of Cepton, is a serial entrepreneur and technologist in optics and electronics. He developed the first optical and acoustical combination technique in semiconductor thin film measurement and holds numerous patents in the field of metrology. Before co-founding Cepton, Dr. Pei founded AEP Technology to develop advanced 3D instruments and pioneered parallel computing in interference microscope. Earlier in his career, he worked as a senior scientist at KLA-Tencor in optics equipment and also led engineering team at Velodyne in advancing the HDL-64 Lidar. Dr. Pei received his Ph.D. in Electrical Engineering from Stanford University.